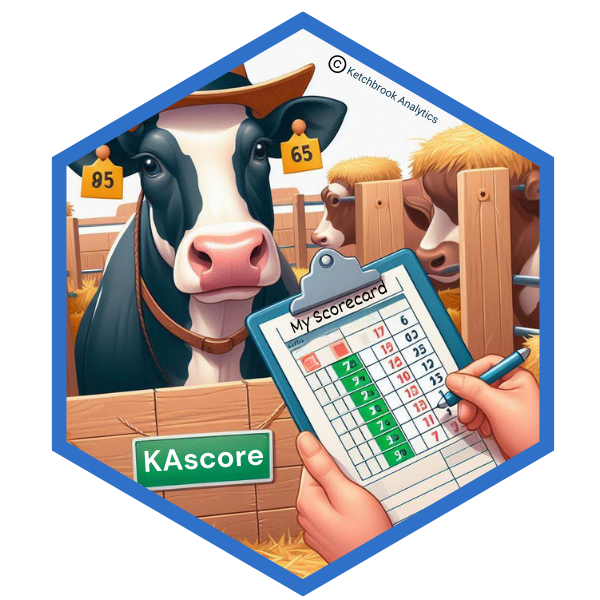
Calculate the Scorecard Points for a Class in an Independent Variable
points.Rd
points()
calculates the number of scorecard points for a
unique class in an independent variable using Weights of Evidence, helping
to build a full "scorecard" of a number of points mapped to each class
in each independent variable.
Arguments
- woe
(Numeric) The Weight-of-Evidence value for a given class of the independent variable
- estimate
(Numeric) The coefficient of the logistic regression model for the independent variable
- intercept
(Numeric) The intercept value of the logistic regression model (where model is trained to predict the probability of "bad")
- num_vars
(Integer) The number of independent variables in the logistic regression model
- tgt_points
(Integer) The target number of points to be used in conjunction with the target odds; see the Details section of
?odds
for more information- tgt_odds
(Numeric) The odds that the
tgt_points
should have; see the Details section of?odds
for more information- pxo
(Integer) The number of points to 'double' the odds; see the Details section of
?odds
for more information- rate
(Numeric) The value to exponentially increase the odds by for the given number of points supplied in the
pxo
argument; see the Details section of?odds
for more information- round
(Integer) The number of digits to round the output score to; default is to round to the nearest integer
Value
A numeric value representing the number of scorecard points for the given class in the independent variable
Details
The tgt_points
and tgt_odds
arguments work together to build a "baseline"
points/odds for your scorecard. For example, a tgt_points
value of 300 and
a tgt_odds
value of 30 would be interpreted as a score of 300 points would
have 30:1 odds of default. See Details section of ?odds
for more
information.
The intercept
value must be from a glm
model trained to predict
probability of "bad". This means that the order of levels in the dependent
variable must be c("good", "bad")
. See ?binomial for more details about
the order in which glm(family = "binomial")
expects the levels of the
dependent variable.
References
Siddiqi, Naeem (2017). Intelligent Credit Scoring: Building and Implementing Better Credit Risk Scorecards. 2nd ed., Wiley. pp. 240-242.
Examples
# Pre-process the data to create WoE features
df <- woe(
data = loans |>
dplyr::mutate(
default_status = factor(loans$default_status, levels = c("good", "bad"))
),
outcome = default_status,
predictors = c(industry, housing_status),
method = "replace",
verbose = FALSE
)
# Fit the logistic regression model
fit <- glm(default_status ~ ., data = df, family = "binomial")
# Extract the model's parameter estimates & intercept
params <- fit$coefficients |>
tibble::as_tibble(rownames = NA) |>
tibble::rownames_to_column(var = "variable")
# Build the scorecard base
card <- woe(
data = loans,
outcome = default_status,
predictors = c(industry, housing_status),
method = "dict",
verbose = FALSE
) |>
dplyr::transmute(
variable = paste0("woe_", variable),
class = class,
woe = woe
) |>
dplyr::inner_join(params, by = "variable")
# Add the points
card |>
dplyr::mutate(
points = points(
woe = woe,
estimate = value,
intercept = params$value[params$variable == "(Intercept)"],
num_vars = length(params$variable[params$variable != "(Intercept)"]),
tgt_points = 300L,
tgt_odds = 30,
pxo = 20L,
rate = 2
)
)
#> # A tibble: 12 × 5
#> variable class woe value points
#> <chr> <chr> <dbl> <dbl> <dbl>
#> 1 woe_industry "" -1.23 -0.991 78
#> 2 woe_industry "beef" 0.231 -0.991 120
#> 3 woe_industry "dairy" 0.0956 -0.991 116
#> 4 woe_industry "fruit" 0.359 -0.991 123
#> 5 woe_industry "grain" -0.410 -0.991 101
#> 6 woe_industry "greenhouse" 0.511 -0.991 128
#> 7 woe_industry "nuts" 0.288 -0.991 121
#> 8 woe_industry "pork" 0.606 -0.991 130
#> 9 woe_industry "poultry" -0.774 -0.991 91
#> 10 woe_industry "sod" 0.154 -0.991 118
#> 11 woe_housing_status "own" -0.194 -0.999 108
#> 12 woe_housing_status "rent" 0.430 -0.999 126
# Calculate points manually
points(
woe = 1.23,
estimate = -0.991,
intercept = -0.846,
num_vars = 2L,
tgt_points = 300L,
tgt_odds = 30,
pxo = 20L,
rate = 2
)
#> [1] 148