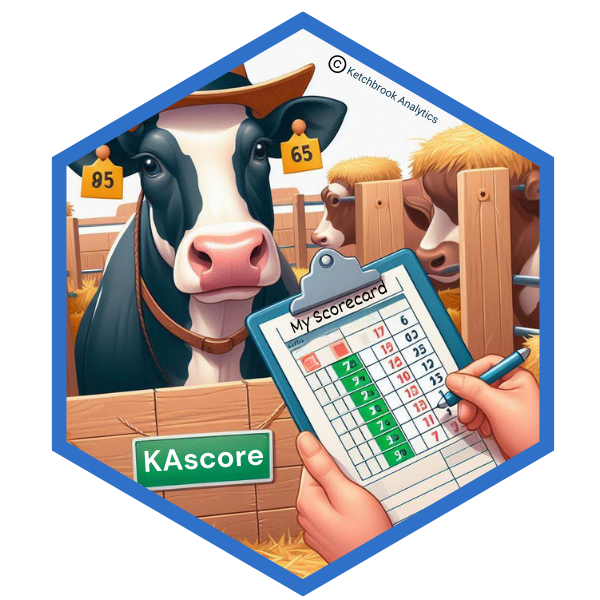
Calculate Weight-of-Evidence
woe.Rd
woe()
builds the Weight-of-Evidence ("WoE")
dictionary of a set of predictor variables upon a given binary outcome.
Usage
woe(
data,
outcome,
predictors,
Laplace = 1e-06,
verbose = TRUE,
method = c("dict", "add", "replace")
)
Arguments
- data
A data frame containing the
outcome
variable and any variables passed topredictors
- outcome
The column variable in the
data
data frame representing the outcome (i.e., the dependent variable indata
); must have exactly 2 distinct values- predictors
<
tidy-select
> The column variable(s) in thedata
data frame representing the independent variable(s) (i.e., "predictor" variable(s)); omitting this argument will select all column variables indata
exceptoutcome
- Laplace
(Numeric) The
pseudocount
parameter of the Laplace Smoothing estimator; default is1e-6
. This value helps to avoid -Inf/Inf WoE values from arising in situations where a independent variable class has only one outcome class in the data. Set to 0 to allow Inf/-Inf WoE values.- verbose
(Logical) Should information on the WoE calculation be printed in the console?
- method
(String) One of "dict", "add", or "replace". Default is "dict", which creates a Weight-of-Evidence dictionary for each unique class across each variable in
predictors
. "add" appends new "woe_*" columns to the input data frame (data
), while "replace" replaces the variables passed topredictors
with the Weight-of-Evidence equivalents.
Details
Negative "WoE" values indicate that there is a higher proportion of
"bads" than "goods" in that particular class of the independent variable,
while positive "WoE" values indicate that there is a higher proportion of
"goods" than there are "bads". This hinges on the assumption that the
levels of the outcome
variable are ordered c("good", "bad")
, otherwise
the inverse is true.
References
Siddiqi, Naeem (2017). Intelligent Credit Scoring: Building and Implementing Better Credit Risk Scorecards. 2nd ed., Wiley., p. 184
Hvitfeldt E, Kuhn M (2022). embed: Extra Recipes for Encoding Predictors. https://embed.tidymodels.org, https://github.com/tidymodels/embed.
Examples
# View the order of levels in `loans$default_status`
levels(loans$default_status)
#> [1] "bad" "good"
# Reverse levels in dependent variable for WoE calculation
df <- loans |>
dplyr::mutate(
default_status = factor(default_status, levels = c("good", "bad"))
)
# Create a WoE dictionary
df |>
woe(
outcome = default_status,
predictors = c(industry, housing_status)
)
#>
#> ── `outcome` variable `default_status` has two levels:
#> • Using "good" as *good* level.
#> • Using "bad" as *bad* level.
#>
#> ── You can reverse this by running:
#> df$default_status <- factor(df$default_status, levels = c("bad", "good"))
#>
#> ℹ See ?woe for further detail
#> # A tibble: 12 × 8
#> variable class n_total n_good n_bad p_good p_bad woe
#> <chr> <chr> <int> <int> <int> <dbl> <dbl> <dbl>
#> 1 industry "" 9 8 1 0.0114 0.00333 1.23
#> 2 industry "beef" 97 63 34 0.09 0.113 -0.231
#> 3 industry "dairy" 181 123 58 0.176 0.193 -0.0956
#> 4 industry "fruit" 234 145 89 0.207 0.297 -0.359
#> 5 industry "grain" 280 218 62 0.311 0.207 0.410
#> 6 industry "greenhouse" 12 7 5 0.01 0.0167 -0.511
#> 7 industry "nuts" 22 14 8 0.02 0.0267 -0.288
#> 8 industry "pork" 50 28 22 0.04 0.0733 -0.606
#> 9 industry "poultry" 103 86 17 0.123 0.0567 0.774
#> 10 industry "sod" 12 8 4 0.0114 0.0133 -0.154
#> 11 housing_status "own" 713 527 186 0.753 0.62 0.194
#> 12 housing_status "rent" 287 173 114 0.247 0.38 -0.430